Machine Learning Algorithms for Face Recognition
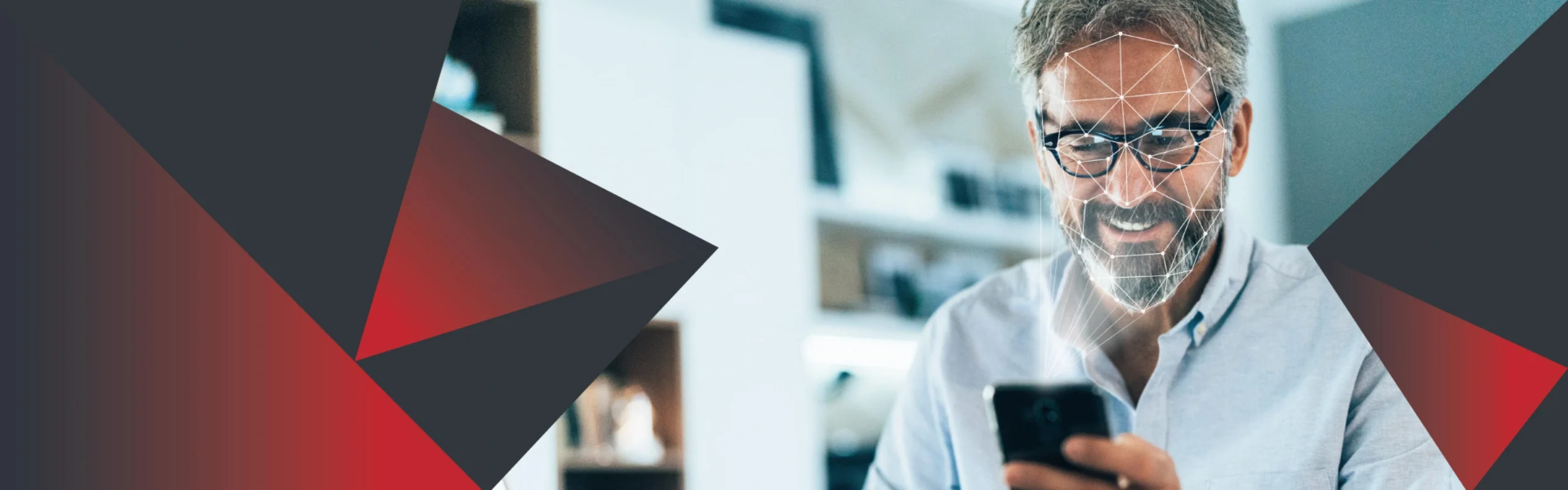
Rapid technological development and adaptation of new technologies cause not only admiration but also controversy. Perhaps never before has this issue raised so much concern about human rights violations. Machine learning technology raises serious concerns in society, especially with regard to face recognition. However, let’s consider the issue seriatim.
The market for Artificial Intelligence itself is huge and it is difficult to find a more promising niche. Even the most skeptical person admits that this is the technology of the future, which directly affects our lives every day even today. According to some reports, the total revenue of the AI market will reach $126 billion by 2025, and this is only the most conservative estimate.
And while many people recognize the potential benefits of AI, Machine Learning, and Deep Learning technologies, the concerns remain. These concerns are primarily related to issues such as the loss of jobs by people and the violation of human rights to privacy.
Today, we’re going to talk about one of these technologies, namely, face recognition. We will tell you in detail how the machine learning face recognition process differs from how our brains do it, dive into the face recognition process and try to break several myths that cause genuine concern among citizens of many countries, including the United States of America.
What is face recognition?
Face Recognition is a technology that is used to remember and identify people by their faces. A particular case of the Face Recognition technology application is user identification through the video power of a mobile device, that is, a camera. So, the user shows his face to the device so that in the future it could recognize this user.
Speaking of identification, such face recognition methods are part of a more general concept – biometric electronic identification. This also includes identification by fingerprint, eye print, and any other biometric indicator.
The machine learning face recognition process usually goes like that: the created ML-powered system remembers the features of an individual’s appearance and becomes able to recognize them in the future, even in the event of changes in appearance, such as a new hairstyle, facial hair, different lightning, and location, etc. In this regard, the machine learning face recognition process is similar to how our brain remembers faces. Someone can do this better, and some less, but we are also able to memorize people’s faces and recognize them in other conditions – light, setting, new details on the face, and so on.
Face recognition technology is most often used to identify and verify users, providing them with access to one or another content, device, service, etc. In the latest conditions of the COVID-19 pandemic, law enforcement agencies in some countries have decided to use face recognition technology in order to track people who are not wearing masks in public places. This decision, like some others related to this issue, caused great concern from the public.
How does face recognition works: the algorithm
In order for the face recognition process to be carried out, it is necessary to have a reading device that can receive video or image data, and a system that will store this data. In the future, the face recognition system will apply mathematical patterns in order to compare the obtained result with the data stored in the database in real-time in fractions of seconds.
This implies the fact that the face recognition process is of two types – primary and secondary (repeatable).
The primary face recognition process includes the following:
the initial reading of the user’s face unique biometric data
saving this data to the database
assigning a specific user identifier to this data
This is a kind of digital onboarding.
A secondary or repeatable face recognition process is everything that will happen after the user’s biometric data has been read and entered into the database. From now on, with each authentication, there will be a process of repeated reading of biometric data to compare it with the data stored in the system for a specific identifier.
As a simple example, we can imagine a mobile application, access to which is provided only through face recognition authentication. So, having opened the application for the first time, the user will allow it to gather and add his biometric data into the system and assign it for his identifiers, for example, credentials – a password and login, or a mobile number. In the future, each time the application is opened, the user will have to show his face in the camera, so that the system, comparing the new data with those already saved in the database, can grant or deny access to the application. It is important to clarify that in order for the face recognition process to occur in real-time, you need an uninterrupted Internet connection.
As for the face recognition algorithm, it includes the following stages. Depending on the preferences of the developers and the chosen technology, these steps can be separate system modules, as well as be part of a single module process.
Face detection. The first step involves the process of identifying a face in an image or video and outlining it with a square. This is necessary in order for the system to compare a specific area with the saved data, and not the entire area that fell into the lens of the device.
Face alignment. The resulting image or video is straightened and resized to the standard size saved in the system. This is done in order to avoid inaccurate comparison and to provide the most suitable face size for comparison.
Facial features extraction. The system reads out certain facial features that will be used for comparison with the stored data.
Face recognition. And finally, by comparing the received data of facial features with those stored in the database, the system may or may not recognize the face. In the case of failed recognition, most often the user is given one more attempt. Sometimes, access is blocked for a certain period of time, which can certainly become a problem. And that brings us to the next section of our article.
Face recognition risks and problems to solve
Despite the obvious advantages that face recognition technology brings, there are a number of problems that industry representatives have yet to cope with. In addition, the introduction of face recognition authentication raises concerns among people regarding the safety of their personal data. Let’s take a look at what risks exist in the industry and what problems need to be dealt with.
Inappropriate collection of data
Yes, face recognition systems serve to increase the level of security. On the other hand, by installing such systems on the streets, in public places, or in any other area, attackers will be able to collect face biometric data and then use it for other purposes. Yes, it is quite difficult to catch the moment in order to capture a person’s face in motion so that this data can then be used for authentication in other systems. Nevertheless, modern cameras and devices are capable of this, and in case of a successful coincidence for the attackers, they will receive data for face recognition without the knowledge of the person at their disposal.
It is almost impossible to completely get rid of such a risk, as you understand. Yes, face recognition software is created and distributed only by trusted machine learning outsourcing or product development vendors and under the supervision of regulators, but it seems obvious that this issue still needs to be carefully worked out precisely from the point of view of legal norms and means of supervision over fraudsters.
Accuracy issue
Although face recognition technology is perhaps the most advanced of all biometric data collection tools, its accuracy leaves much to be desired. Of course, things are much better today than they were a few years ago. However, the system can still be tricked. Deepfake technology, which uses a deep learning approach to replace a person’s face, creates an effect that is almost indistinguishable from reality. All this is an additional contender for the widespread or mandatory introduction of face recognition technology since the accuracy of such a system and its AI fraud detection still leaves much to be desired.
Lack of access in an emergency
Any advanced protection system is really good in a calm environment. However, as we have already found out, there is a problem with the accuracy of face recognition systems. What happens if in an emergency situation an authorized person cannot access his device, car, or building? Simply put, this problem can be described as a statement: you cannot give the face recognition system too much power, because then it can limit us in our freedoms. The same as with any other artificial intelligence or machine learning solution.
Moral and ethical issues
One of the most acute and hotly debated issues in the context of Face Recognition technology is the ethical issue that raises conservatives about human rights violations. Many people are deeply convinced that their governments will use face recognition technology in order to strengthen their control over the movement of citizens and in one way or another limit their freedom. And although this thought has the right to life, do not forget that our smartphones are also capable of transmitting geolocation data.
The main problem in this matter is that any new technology is perceived by society with caution and face recognition is no exception. Most people aren’t ready to embrace the new technology and believe that it will only be used to improve their security, which is justified. And although everyone has long been accustomed to the fact that their faces can be captured in public places thanks to the cameras installed there, not everyone is ready to put up with the fact that face recognition technology will allow specialists to recognize and analyze their faces.
Business concerns
Of course, users’ distrust of technology has an effect on businesses as well. Understanding all the controversy surrounding face recognition technology, many businesses refuse to integrate it into their processes or invest in face recognition solutions development. All this undoubtedly slows down the process of technology development, although this effect does not have its critical mass and the arrival of face recognition in our everyday life will take place one way or another. The only question is when exactly.
Face recognition software and legal rules & principles
Of course, in order to cope with most of the problems listed above, an initiative from legislators is needed. Such a serious technology as face recognition should be used strictly within the framework of the current legislation so that both business and customers can defend their rights in court if necessary. In addition, the introduction of legislation will calm businesses and investors, which will open up new ways for entering the face recognition industry.
As far as the European Union is concerned, everyone who uses or produces Face Recognition software is obliged to follow the main law in the EU regarding security and data protection – GDPR (General Data Protection Regulation). This law is versatile and is constantly being supplemented depending on the pace of new challenges and technologies’ emergence. In the United States, the situation is moving at a slightly slower pace.
Of course, the peculiarity of the United States is that each individual state is obliged to develop and vote in the Senate its own law regarding the protection of personal data and the obligations that manufacturers and users of face recognition software must follow.
It all started when California, using the GDPR as an example, developed its CCPA (California Consumer Privacy Act). In this document, the experts expressed all the best that could be applied from the GDPR and updated some of the norms in accordance with American practice. It would not be an exaggeration to say that this action from the California authorities really inspired other states to pass similar legislation on their territory.
So, very soon (at the beginning of March 2021) Virginia adopted its CDPA (Consumer Data Protection Act). Washington, Connecticut, and Texas were supposed to be the next states to vote for their laws, but the process is going hard. California has really inspired other states with its example, but in each case, the process is delayed. Thus, a vote on the law in Washington did not pass voting, while in Connecticut and Texas, these laws were postponed for consideration by special commissions on data protection. This is done so that the law on such a sensitive topic as the consumers’ rights and personal data has a final and complete form without any pitfalls.
Nevertheless, despite all the difficulties, the process doesn’t stand still. In 2021, New York and Oklahoma voted for their laws following California’s example. Illinois is not far behind with its Biometric Information Privacy Act (BIPA). In all these states, it is already possible to legally produce and use face recognition technology without fear of any violations or future bans. Therefore, if you are looking for the best place in the United States for your face recognition project to start, these states are the ones that will fit you most.
Deep Learning and Face Recognition
It wouldn’t be an exaggeration to say that Deep Learning, as part of the broader concept of machine learning, was at the origin and the first applications of Face Recognition solutions.
The year 1987 and the Eigenfaces algorithm can be called the beginning of the successful history of Face Recognition Deep Learning. Attempts to apply deep learning understanding to create a face recognition algorithm have been made before, but Eigenfaces can be considered a truly successful first working solution. The algorithm of the solution is best described on its wiki page:
The eigenvectors are derived from the covariance matrix of the probability distribution over the high-dimensional vector space of face images. The eigenfaces themselves form a basis set of all images used to construct the covariance matrix. This produces dimension reduction by allowing the smaller set of basis images to represent the original training images. Classification can be achieved by comparing how faces are represented by the basis set.
Deep Learning solutions developed in a similar way throughout the 90s and early 2000s. The next stage can be safely considered AlexNet and its incredibly successful presentation at the ImageNet Large Scale Visual Recognition Challenge in 2012. The published documentation of AlexNet is widely regarded as one of the most influential documents about Deep Learning for Face Recognition. Unsurprisingly, it has generated a new influx of interest and investment in Deep Learning’s methods for face recognition research. Over the next 4 years, the capabilities of new algorithms first caught up and then surpassed human indicators. The result of these studies were four main algorithms that showed the best results – DeepFace, DeepID, VGGGFace, and FaceNet.
Some of these algorithms are widely used today to create pre-trained deep learning face recognition algorithm. The pre-training method is one of two approaches to using deep learning for face recognition.
When making a choice in favor of the pre-training method, the developers turn to well-known models, such as the above-mentioned DeepFace or FaceNet. The choice in favor of this method is due to the fact that these models no longer need to be filled with millions of images and trained, they already contain algorithms for face recognition tasks. These algorithms can be customized and adjusted for specific needs, but in general, the system is configured and ready for analysis.
In contrast to this method, you can develop a deep learning face recognition system from ground zero. Of course, this method requires more time and resources. On the other hand, this is exactly what you should do in a situation if you need a face recognition system with unique features.
When developing a deep learning solution for face recognition, it is worth considering such parameters as choosing the right type of neural network for development, choosing the right architecture for the neural network, and the power of your hardware.
It is crucial to perform face recognition using neural networks. What’s more important is that to create such algorithms specifically for face recognition tasks, it is better to use convolutional neural networks (CNN). This is explained by the fact that the CNN structure represents itself as a chain of neurons, in which the first neuron as the output can serve as an input for the next neurons, and so on, thereby simulating the connections that are inherent in the human brain. When a large number of such neurons are connected to each other and layered, this is already a model of a neural network.
From the point of view of specifically face recognition tasks, there are several methods to improve the performance of a neural network.
The first is knowledge distillation. So, in order to speed up the process and increase the efficiency of the neural network, two neural networks are created – a large and a small one. A large neural network teaches a small one, which ultimately leads to the fact that a small neural network demonstrates faster work with the same recognition efficiency as a larger one.
Another good method is called transfer learning, and it includes training a neural network on a specific, smaller dataset. For example, in order for the neural network to learn faster and recognize faces more efficiently in the future, you can first train it on a smaller dataset, eg., instead of all types of faces, you can select Asian faces only.
The main thing to remember is that in order to create face recognition using deep learning, as well as to train and obtain the result of the operation of such a neural network, it is necessary to have a resource with great computing power. This will directly affect not only the accuracy of the algorithm but also the speed of outputting the result, which is also critically important.
Face recognition using machine learning & Python
Python is by far the most popular programming language among developers for building machine learning solutions. According to some reports, about 60% of developers involved in providing machine learning services are using Python. This advantage is understandable, since, in addition to the fact that Python is relatively easy and understandable to learn, it also boasts the most diverse set of libraries and tools sharpened for machine learning development, for example, such popular libraries as Numpy, TensorFlow, or PyTorch.
In the context of face recognition in machine learning questions, the most commonly used AI concept is Computer Vision. At its core, this AI algorithm for face recognition describes how exactly a computer sees and recognizes the details of an object in an image or video. What our brain performs in a second on a subconscious level, the computer does in several stages: it recognizes an object, its shape, its color, its appearance, and so on.
In addition to the libraries mentioned above, there is another extremely popular solution for performing computer vision tasks on Python – OpenCV. It is an open-source library that runs on Python & R languages and supports all major platforms including Windows, Linux, and macOS. The advantages of OpenCV are obvious: it’s free of charge, it’s fast (the library is written in C/C++), and it requires less hardware power.
Installing OpenCV does not cause any problems and the speed of this library is due to the fact that, unlike many other solutions, OpenCV uses the cascade method to check the classifiers, instead of going the classic path from the upper left corner of the image to the moment when the face will be detected. Due to this cascade method, the face detection algorithm is faster than in other libraries.
The essence of the cascade face detection using machine learning is that for each individual block of the image, OpenCV performs a quick and small test. If the test is successful, a more detailed test is started, and so on, until the face is detected. One way or another, the library will need about 50 cascade tests to detect the face, but this is still faster. This is why OpenCV is faster and requires less RAM from the computer, and why this library is probably one of the best face detection algorithms. Some developers claim that they can write a face recognition algorithm in OpenCV and fit it into 25 lines of code.
In general, be it OpenCV or another library, Python certainly has the greatest potential for creating the best algorithms for face recognition tasks.
Bottom line
When it comes to face recognition, it’s hard to avoid controversy. On the one hand, the indignation and public concern, especially in the United States, is understandable. On the other hand, watching how government regulators begin to understand the issue and clarify the legislation, more and more people start to support face recognition technology. The prospects and the benefits that face recognition solutions can bring to our lives cannot be denied. Nevertheless, answering the question put forward in the title of this article, we would like to say that it is still difficult to give an answer. Face Recognition is definitely a controversial technology, as well as promising in terms of its application. We would like to believe that opponents and followers of technology will be able to find a compromise in order to prepare society for the introduction of face recognition technology.
FAQ
Deep Learning, being part of the more general machine learning concept, is the progenitor of the entire face recognition industry. Data Scientists and developers use the deep learning method to create face-recognition algorithms based on the method of block-by-block checking of an image or video. Some of the best examples of Deep Learning applications in face recognition are algorithms such as DeepFace, FaceNet, and DeepID.
The most popular and well-known machine learning algorithm for face recognition is the Viola-Jones algorithm. It detects photos in several stages: feature definition, feature assessment, feature classifier definition, and classifier cascade check. For example, the OpenCV library works exactly like that.
Face Recognition is an AI-based mechanism that exists to determine, recognize and classify faces depicted in images or videos, by applying the power of neural networks trained on a large number of different images.
There are many different languages that are being used to create face recognition solutions, but Python is by far the most popular one. This is due to the relative ease of learning, the presence of a large number of open-source libraries (OpenCV, TensorFlow, Numpy, etc.), and the computing power of solutions created on Python.
Build your ideal
software today
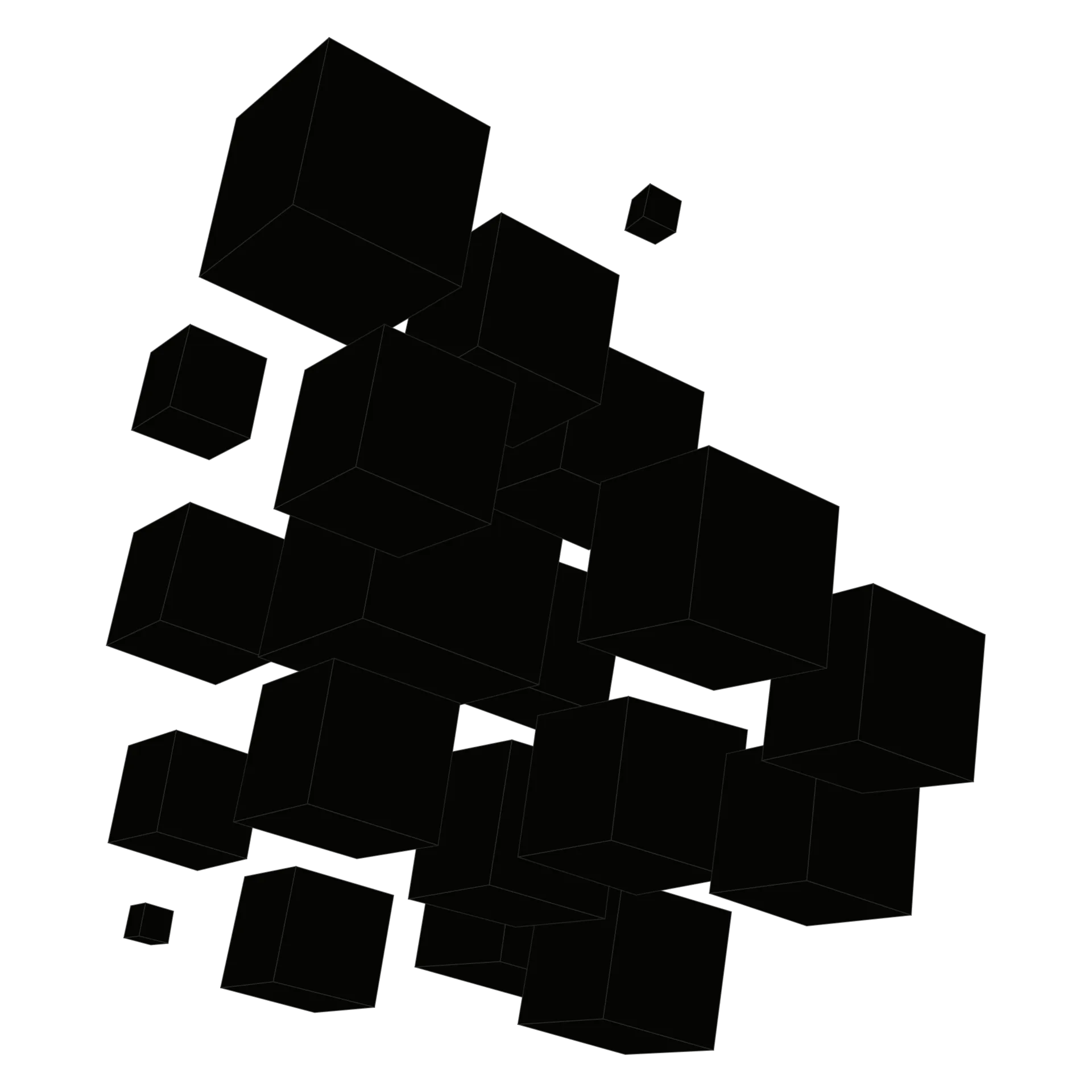